Parcus Group can develop comprehensive data analytics based telecom customer churn prediction models which are built on corporate or consumer customers data.
The models assess all customers and aim to predict churn and loyalty behaviour based on the analysis of demographic data, customer purchases history, service usage and billing data. All characteristics and transactions are analysed, ranked and modelled to create customer or segment loyalty profiles.
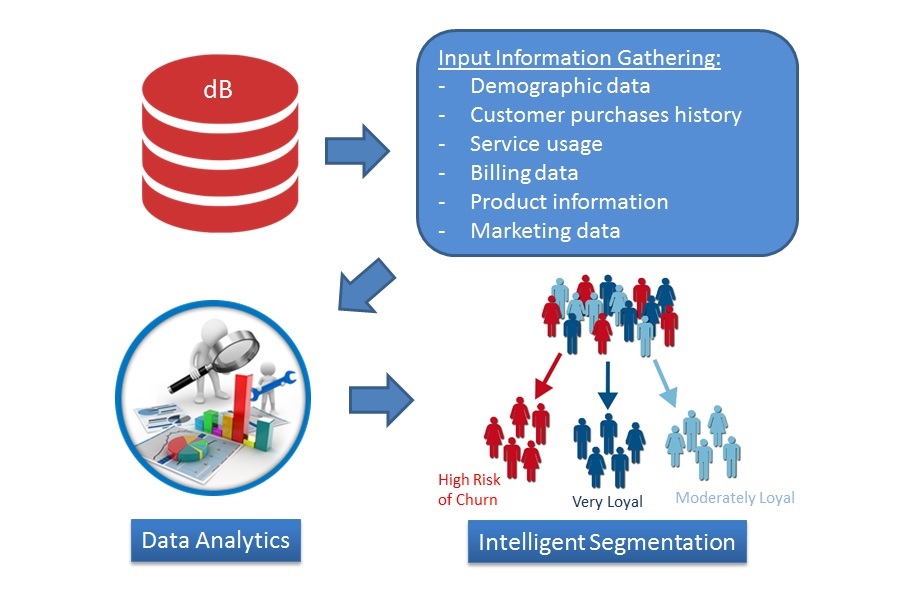
Some of the information required to develop comprehensive and accurate churn models will include:
- Numbers of total customers a carrier has
- Percentage of customers that currently churn every year including the definition of churn eg. dormant for x months or only actual disconnections?
- Ideally at least 1 year of data which can be used for modelling (longer data history and greater data quality will ensure accurate and powerful models, though custom solutions may be designed for every dataset)
- Some or all of the following key information variables:
- Customer demographics data (postcode, income, occupation, address, marital status…)
- Customer purchase history (buy location/channel, spend, refills, number of services, buy date, cancel date…)
- Service usage data (number of calls, in/out, SMSs, minutes, data usage etc…)
- Billing data (total amounts spend, spend breakdown by voice, SMS, data, payment history on time, payment delays, missed payments etc…)
- Product information (product mix, pre-paid or post-paid, bundles.
- Marketing data (existing segmentation views, competitors, competitor campaigns and impact on churn, competitor pricing…)
Also it is advisable to consider the following questions in advance while planning for churn models development as this will assist with project scoping and expedited delivery.
- How does the telecom carrier intend to use the churn predictions ie. for improved marketing segmentation, for targeted marketing campaigns etc..?
- How does the telco intend to implement the churn model eg. as a standalone spread-sheet or database or integrate it with you other systems?
- Does the company have any internal Customer Value Management (CVM) team and data analytics skills that can be used for regular model updates or on-going churn modelling support is required?
- Expectations around project deployment time and budgets?
Construction of Parcus telecom customer churn prediction models leverages a mixture of quantitative and qualitative statistical techniques including multi-variable logistic regression, an industry standard modelling technique as well as some of the newer methodologies such as machine learning, decision tree based modelling, gradient boost models and others.
Based upon the resulting loyalty or churn prediction score a predetermined marketing and segment strategy can be recommended for each customer, group of customers or a whole customer segment.